How to utilize data analytics in project management
Guest Post
April 18, 2024
There’s no escaping it: in the modern world, data is king. As technology advances, we’re seeing more and more ways of collecting and analyzing data. This gives us actionable insights, powering our marketing, customer service, and sales.
But data isn’t just valuable for external purposes. Businesses are increasingly using data internally, including to improve project management processes.
If you don’t understand a thing about data analytics, don’t worry. This guide will explain everything you need to know, from the connection between data and project management to how you can implement analytics effectively.
What is data analytics in project management?
Unless you’re AI, raw data isn’t that useful. To the naked eye, it looks like a string of unintelligible numbers. That’s why data analytics tools come in handy. They collect, unpack, and present data in a way that makes sense. Usually, they do this through user-friendly interfaces with plenty of visualizations.
Whether you work in advertising or HR, these tools can be transformative for every aspect of a business. Uncovering patterns and trends in large datasets can give you insights that you wouldn’t otherwise have access to. With this information, you can then optimize everything from operations to budgets.
What are some types of tools used?
In project management, various types of tools are utilized for data analytics to track progress, monitor performance, and make informed decisions.
These are just a few examples of tools used in project management for data analytics. Depending on the specific needs and requirements of the project, different combinations of tools may be used to track progress, monitor performance, and make informed decisions throughout the project lifecycle.
Kanban boards
Project management teams often leverage specialized project management tools that come with Kanban boards to streamline workflow processes and visualize task progression. Kanban boards allow teams to visually track the status of tasks, identify bottlenecks, and prioritize work items, ultimately improving efficiency and project delivery.
Burndown charts
Burndown charts are graphical representations of work remaining versus time. They are commonly used in Agile project management to visualize progress and predict when all work will be completed. By analyzing burndown charts, project managers can identify trends, forecast project completion dates, and make adjustments to optimize project delivery.
Gantt charts
Gantt charts are bar charts that represent project schedules, with tasks listed along the vertical axis and time along the horizontal axis. Gantt charts provide a visual representation of project timelines, dependencies, and resource allocation. By analyzing Gantt charts, project managers can track progress, identify critical paths, and adjust schedules as needed.
Dashboards
Dashboards are interactive data visualization tools that consolidate and display key performance indicators (KPIs), metrics, and project data in a single interface. Project management dashboards provide real-time insights into project health, performance, and status. By analyzing dashboard data, project managers can monitor project progress, identify issues, and make informed decisions.
Reporting tools
Reporting tools generate standardized reports and ad-hoc queries to extract insights from project data. These tools often offer customizable templates and formats for various types of reports, such as status reports, progress reports, and risk reports. By analyzing reports, project managers can communicate project status, trends, and performance metrics to stakeholders and make data-driven decisions.
Resource management tools
Resource management tools track the allocation, availability, and utilization of resources (e.g., personnel, equipment, budget) throughout the project lifecycle. These tools provide insights into resource capacity, demand, and allocation efficiency. By analyzing resource data, project managers can optimize resource allocation, mitigate risks, and ensure project success.
Predictive analytics tools
Will you meet your targets based on current working patterns? Are you likely to go over your budget? Predictive analytics tools use statistical algorithms and machine learning techniques to forecast future trends, outcomes, and performance metrics based on historical data. By analyzing predictive models, project managers can proactively manage risks, allocate resources effectively, and improve project outcomes.
Benefits of data analytics for project managers
Data analytics tools come with a lot of functionality. But how can that benefit your processes? Here are some of the crucial reasons for using data analytics in project management.
Data-driven planning and forecasting
One of the key areas where data analytics can significantly benefit project management is in planning and forecasting. By analyzing historical project data, such as timelines, resource allocation, and budget utilization, project managers can develop more accurate project plans and forecasts. Predictive analytics techniques enable project managers to anticipate potential bottlenecks, resource constraints, and project delays, allowing them to proactively address issues before they escalate.
Performance monitoring and KPI tracking
Monitoring project performance and tracking key performance indicators (KPIs) are essential for evaluating project progress and identifying areas for improvement. Data analytics enables project managers to track KPIs in real-time, allowing them to assess project health and performance metrics such as cost variance, schedule variance, and quality metrics. By leveraging data visualization tools and dashboards, project managers can communicate project status and performance metrics effectively to stakeholders, enabling transparency and accountability throughout the project lifecycle.
Create more streamlined projects
As any project manager knows, projects can run into numerous bottlenecks. Imagine you’re overseeing a project to improve the efficiency of your customer support. Say, for example, the plan is to introduce click to dial software, but on the day of implementation, you’re struck by server issues. These delays can hamper your ability to meet your goals.
Project management data analytics tools look at all the processes involved in your project. Using the aforementioned machine-learning technique, they can spot inefficiencies. They’ll then notify you about areas that you can improve and even take action to address these.
Ensure effective resource allocation
Projects can consume lots of valuable resources. Whether it’s cash, time, or materials, you can find your list of resources running low fast if you’re not careful. So, how can you ensure you’re spending resources effectively? BI tools can help by identifying inefficiencies and suggesting improvements.
For example, let’s say your teams are investing lots of time in manually finding customer emails. Thanks to your BI analytics tool, you spot this inefficiency and decide to invest in an email lookup tool. Now, your team can find customer emails much more quickly.
By implementing automation tools that enable your team to automate data entry and quickly find information, you free up valuable time that can be focused on more strategic aspects of project management.
Improve risk management
Risk plays a big part in project management. Issues can range from minor problems, like running slightly behind schedule, to full-blown catastrophes, such as large-scale data loss. One sure thing is that anticipating and managing these risks is critical to your project’s survival.
But sometimes, the risks aren’t clear. A crisis can strike your project from nowhere, leaving you picking up the pieces.
With access to high-quality data, you can overcome these hurdles. Analytics software looks at past and present trends to identify potential risks. You can’t avoid everything. However, you can ensure you base every decision on data-driven insights, minimizing risks and keeping your project running smoothly.
Boost team members’ performance
Every team member has strengths and weaknesses. For some, those weaknesses might be time management; for others, it’s teamwork. By ironing these out, you can create a more efficient team and even a more pleasant working environment.
Analytics can monitor employee behavior and spot where they’re struggling or falling behind. Once these issues are identified, you can help employees overcome them by creating focused training and providing them with tailored support.
This point comes with a caveat, though. Analyzing employee performance has ethical implications. Not everyone on your team will be keen on being monitored. If you go down this route, be transparent with team members. Try to be as noninvasive as possible and explain how data will help them and the wider team.
Additionally, implementing effective team collaboration strategies is vital for project success. Encouraging open communication, fostering a culture of trust, and utilizing collaborative tools can enhance teamwork and productivity.
Also, data analytics facilitates the connection of hybrid teams, fostering collaboration between on-site and remote members. This is achieved by providing a centralized platform accessible to all team members, allowing them to access real-time project data, collaborate on tasks, and communicate effectively regardless of their physical location.
Challenges of using data analytics in project management
- Reliance on technology: Data analytics is great, but it shouldn’t replace the human element of project management. Instead, it should be a piece of the puzzle. To make the most of your data-driven insights, they need to work in tandem with the people, processes, and technologies of your project.
- Managing data: Many businesses work with unstructured data from isolated sources. Not only is this incredibly inefficient, it can result in errors and hamper decision-making. It’s important to follow a data quality management framework to effectively handle complex data, detect errors and invalid data points, and stay compliant.
- Communicating data-driven insights: BI tools can provide a lot of useful insights. But the way you communicate these with colleagues or stakeholders is critical if you want to make those insights actionable. Learning how to share information effectively takes time and some colleagues may need convincing.
Data analytics best practices
If you’re ready to start gleaning insights from data, great! As you do, though, bear these points in mind.
Create SMART goals
Why are you gathering data? It’s a simple question, but might be more difficult to answer than you think. If your answer is ‘to create more efficient projects’, that’s likely too broad to be useful. You need to center your data collection around SMART goals.
Don’t just think about the long-term objective of your project; sub-goals should also be considered in each step of the journey. When setting your goals, use the smart framework:
- Specific: Each goal needs to be highly specific to what you want to accomplish in your project.
- Measurable: You need to be able to track progress, so attach goals to key performance indicators (KPIs).
- Achievable: Your goal might sound great, but can you achieve it? Be realistic rather than idealistic.
- Relevant: Your goal should sit within your wider, long-term objectives.
- Time-bound: How long do you have to complete your goal? Again, think of this in the context of your wide project deadline.
Choose analytics tools carefully
There are a lot of project management BI tools out there.
Many have similar functions, but each will differ slightly. Finding an option that fits your needs is essential for getting the most out of your data.
Aim for a solution that offers some of the following features:
- Centralized data: Information for all your different data sources should be available in one place.
- Integrations: Can you connect your chosen tool with other tools you’re already using, such as a project management tool or a CRM?
- AI-powered insights: As mentioned, modern analytics tools can make use of AI-powered machine learning to offer smart insights. Look for software that offers predictive analytics to ensure projects are headed in the right direction.
- Project performance tracking: You should be able to monitor your progress broken down into individual goals.
- Detailed visualizations: Visuals help to make data more accessible, so your chosen tool should include a variety of visualization options, from bar and line graphs to waterfall charts and heat maps.
- Customizable dashboards: Dashboards should provide quick access to the data that is most important to you.
How to choose the best data source
Once you’ve chosen your analytics tool, you need data. Project management tools are a great source for this as they will already be collecting data relating to key project areas.
This includes:
- Budget
- Timescales
- Activity dependencies
- Time spent
- Percent completion
- Resource allocation
Remember, you might need to match or cross-reference data to other sources in the organization. Your central HR repository can be a useful source for resource information, skills, and experience.
Similarly, your commercial database will have details of contracts and commercial relationships, while your CRM will hold customer data. Depending on your needs, your analytics could require a blend of data from these and other sources.
If you’re lacking historical data, don’t worry. There are plenty of sources that can give you the quality data that you need – the key is to select the best source for your requirements.
External data sources can be beneficial, especially for comparison or best-in-class cases. Sources like the Project Management Institute have high-quality data to power your analytics.
Of course, your own data will always be the most valuable. If you have lots of historical data, great; use this to support your predictive analytics. If you’ve yet to begin your data collection operation, you can configure your BI tool to get the info you need.
How to onboard your team with new data analytics software
Introducing analytics to project management can be a learning curve. Not only do team members need to get to grips with new software, they also need to learn a new approach.
At first, some employees may be uneasy – people don’t generally like change. It’s your job to convince them that analytics will benefit the team.
Just as you might have to give an API definition if a project involves programming, you’ll need to give a low-down of your new analytics software. Be patient – some employees may take longer to adjust. Provide training on the importance of data-based decision-making, and ensure the team understands the value of data.
It might take time and effort now, but supporting your team during this period will pay dividends in the long run.
Always clean data
The concept of cleaning data might sound odd. But if data isn’t properly cared for, it can contain issues like duplications, cardinalities, and inaccurate entries. These problems all throw off your insights. While you may think your data-driven information is useful, it’s leading you astray.
Clean data is an essential factor if you’re interested in making the most of future AI advancements. Quality data is key for training AI and improving machine learning.
And don’t think these issues are uncommon. As we said, many project managers will take data from different sources. This means you’re likely to get duplicate or out-of-date entries. A regular cleaning – once a year for small businesses and every three months for larger enterprises – can help you avoid these problems.
Utilizing an event checklist can be invaluable to ensure thoroughness in managing project tasks and deadlines. This tool helps in systematically organizing and tracking all necessary steps and activities required for successful event execution, minimizing oversights and maximizing efficiency.
Present your insights carefully
As mentioned, one of the challenges of project management data analytics is presenting your data.
Chances are, you know how valuable data-driven insights are. However, some team members may need convincing. You won’t accomplish this by providing confusing statistics and numbers in a haphazard way.
Effective data analysts share insights in the context of a wider story, one in which the recipient is the main character. By presenting data in a way that is relevant to colleagues or stakeholders, you’re far more likely to get your message across and create a path for making improvements.
So, when presenting data, ask yourself the following:
- Which data is relevant to my audience?
- Does this data answer the audience’s questions and concerns?
- Do my insights help overcome project barriers?
- What data visualizations will support my storytelling?
Final thoughts
As technologies like machine learning continue to advance, data is only going to become more important in project management. To avoid being left behind, now is the time to start making use of data.
By leveraging project management software like Backlog, you can take advantage of reporting and analytics features to track project performance, monitor progress, and make data-driven decisions. Once you’re set-up, there’s no looking back. Embrace the data-powered future of project management.
Author bio
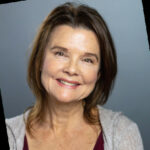
Cory Plachy is the senior marketing and communications manager at Convoso. Adaptable and naturally curious, Cory channels years of content creation, marketing, and sales experience into the world of SaaS communication. She has written for domains such as Wordable.io and PushFar. Connect with Clory on LinkedIn.